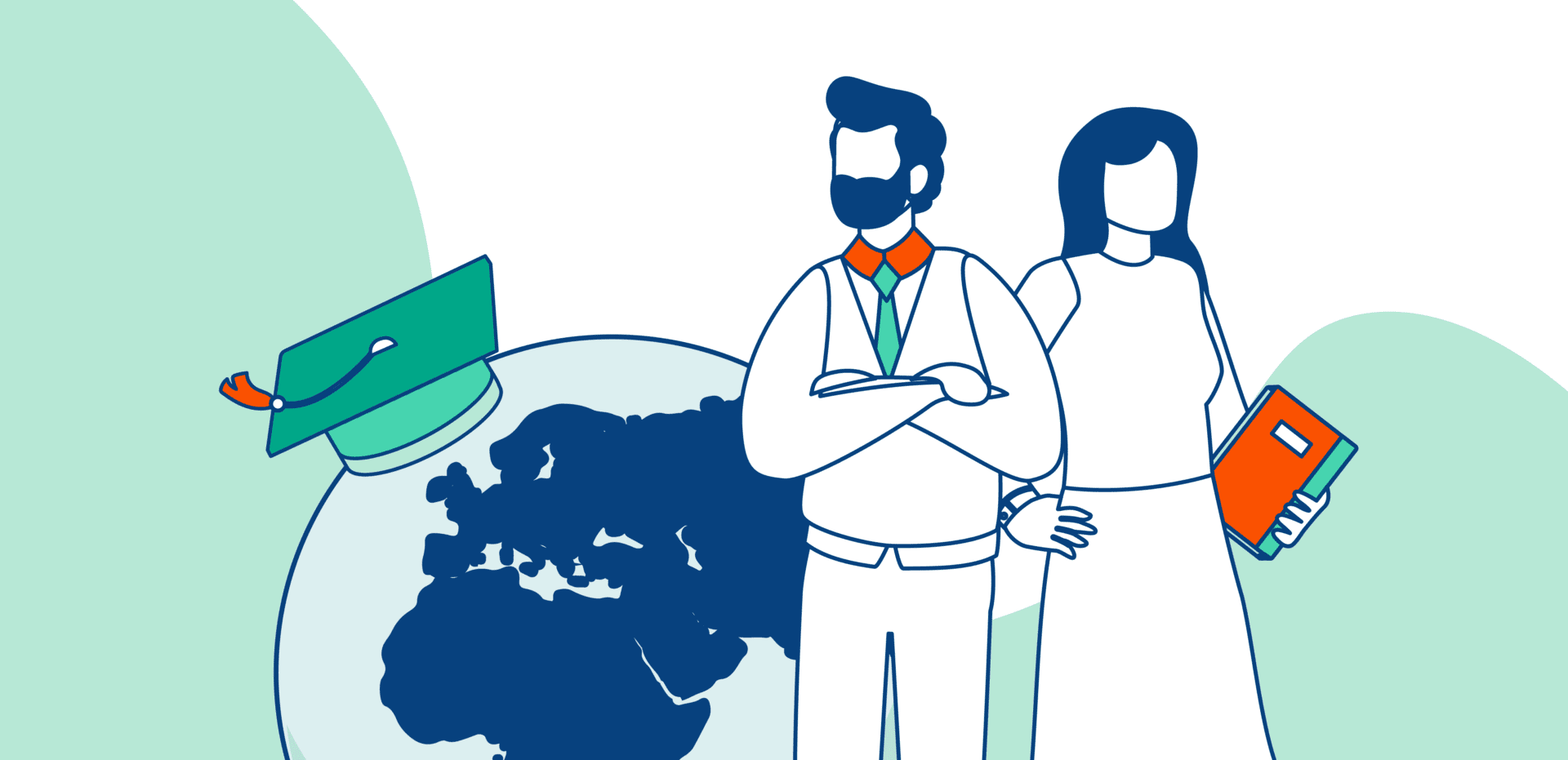
Between April and November 2024, we ran a series of workshops across the globe to better understand what educators wanted from artificial intelligence (AI). Much of the focus of the last two years has, rightly, been on how students are using generative AI capabilities. An equally important part of the picture is how educators perceive AI in their work. What do they see as the right amount of AI, if any.
The workshops were held at Times Higher Education’s Digital Universities events in Exeter (UK), Bali (Indonesia) and at our Inspera events in Brisbane, Sydney, Melbourne, Adelaide, Oslo and London. At each event we provided the audience with a series of AI capabilities and asked if they would use them. Using the principle of Trustworthy AI we set out an important caveat with each scenario: an assumption that the AI capabilities met the ethical, data privacy and protection policies of the institution. We are aware that without meeting these requirements, AI capabilities won’t be used.
In analyzing the data from almost 300 respondents we are also aware that the cohorts predominantly comprised those with an interest in emerging technologies through their attendance at events with a tech slant. A wider study of academics might provide different sentiments. Workshops were conducted using Mentimeter with the audience answering anonymously. Respondents were asked to indicate their sentiment using the five point Likert scale.
As well as presenting the results to you in this post, it formed a useful background to our Trustworthy AI paper.
AI in Education: Key Themes
How Do You Feel About AI?
Respondents were asked a free text question to summarize their feelings about AI in education. Three predominant themes emerged. First, that AI in education was inevitable. Further discussion revealed that this was split between resigned inevitability and an accepted part of technological progress. Many respondents were keen to add that they wanted to shape how AI was deployed in their institution. Second, that AI, used correctly, had great potential but that potential differed depending on cognate subject, cohorts and context. Third, that the rapid advancement of AI was potentially a little scary. The ‘newness’ of the technology, change velocity and that there was the possibility of it being used incorrectly, all played a part in their sentiment towards it.
A number of themes emerged from the questions we asked. They highlighted both the potential and concerns educators have in the use of AI, particularly generative AI. They demonstrate the deep engagement taking place across the world with technology that is emerging in possibilities and in widespread use.
Can I Edit the Output?
A recurring theme was the demand for AI-generated content to be editable. Educators want control to ensure it aligns with their context. This reflects a broader sentiment that AI should serve as a tool to enhance human capabilities, not replace them. The ability to refine and adjust the output is a necessity to maintaining quality.
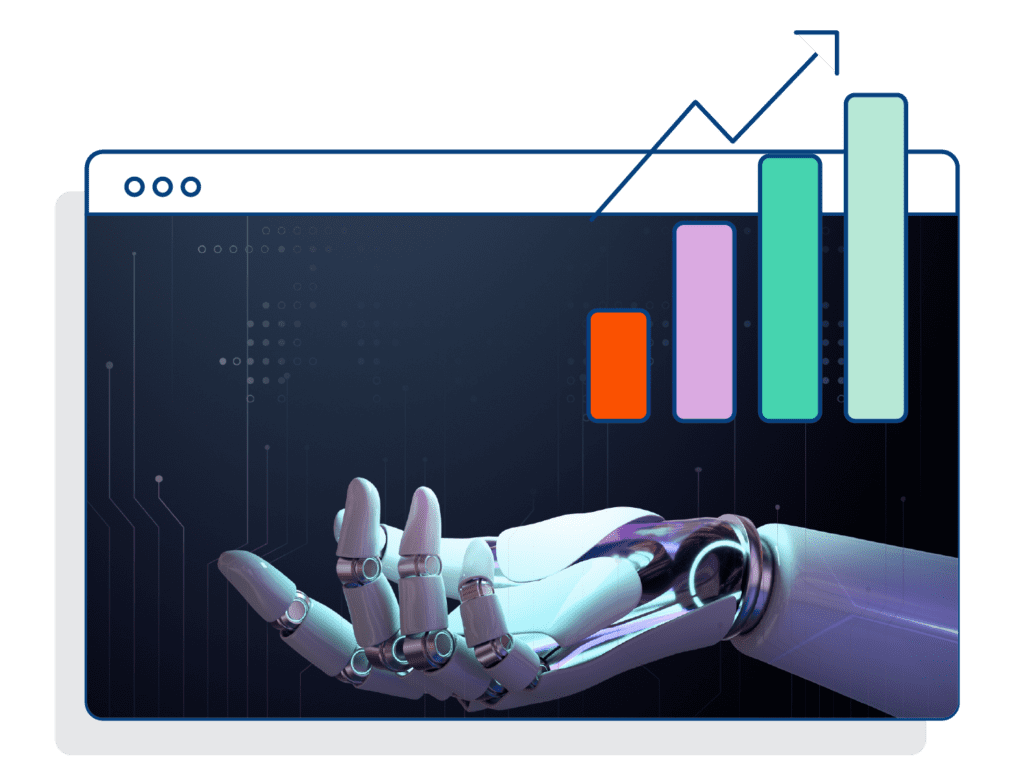
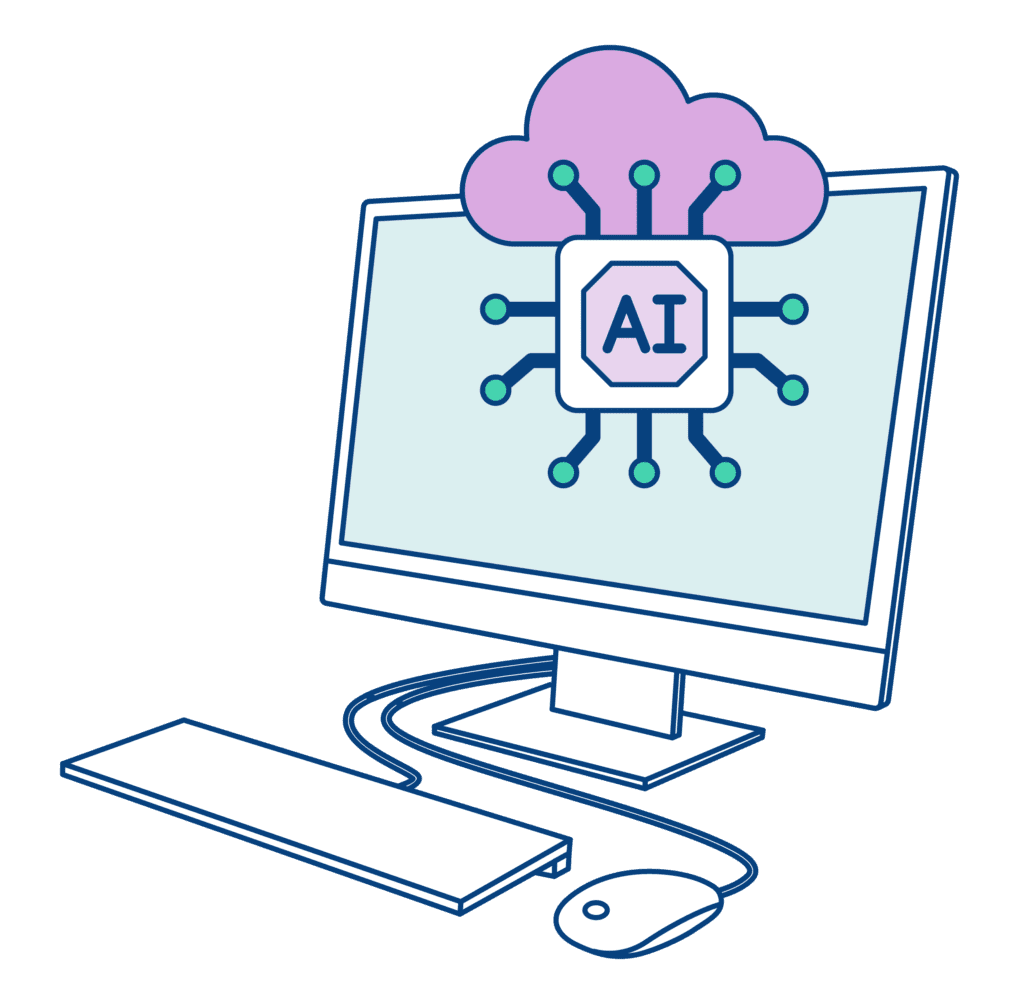
AI on My Content, Not AI Working on AI
Where AI might be used to generate content, there was a strong preference for AI to generate from human-created content rather than generative AI on generative AI. The concern was twofold: insufficient human input in an area where human input was critical, and the potential for inaccuracies to be compounded. Instead, they want AI to work directly with their own content and within their learning, teaching and assessment to drive relevance and reliability.
Transparency in AI Processes
Educators want clarity on how AI processes their content. A number noted that they need a better understanding of how AI goes beyond existing technologies like machine learning or traditional computational matching. What distinguishes AI from other digital tools, and how does it add value? This transparency is critical for building trust and ensuring that AI is perceived as an advancement rather than a black box. Further discussion clarified that a desire not to become an expert on the inner workings of AI, but an expert on how AI works on their content.
Quality of AI-Generated Metadata
Metadata creation is one area where educators see potential for AI, but they had questions. They are open to AI generating metadata for their content and are keen to see the quality and reliability of such metadata. Will AI-generated metadata be accurate, meaningful, and contextually appropriate? The initial results are likely to color its future use.
Reluctance Toward AI-Generated Metadata on AI Content
Interestingly, educators are far less comfortable with AI producing metadata for content that was itself generated by AI. This highlights a general concern about the compounding use of AI, where one AI process builds on another. For many, this introduces an unacceptable level of abstraction and uncertainty. A strong preference was indicated to limit AI’s involvement to scenarios where it interacts directly with human-created content.
Protecting Pedagogy
Perhaps the most emphatic point raised by educators is their desire to keep AI from inappropriately encroaching on their teaching roles. Teaching is a fundamentally human activity, relying on inherent knowledge and skills to respond to individual students. While AI can support tasks or enhance efficiency in other areas, educators want clear boundaries to ensure that AI does not diminish the human connection at the heart of education. Concern too was expressed about how students might negatively value interaction that felt (even if it was not) machine generated. Assessment feedback being a prime example.
Findings From Our Questions
During the workshops, educators were asked to indicate their level of agreement with the following statements using a five-point Likert scale.
-
-
- I’d use AI to generate non-MCQs
- I’d use AI to tag my (human generated) questions to create a taxonomy for an item bank
- I’d use AI to ‘dry run’ an assessment I have written to give me a range of possible student answers
- I’d use AI to analyse data on my assessment questions and student responses
- I’d use AI to generate follow-up activities for students based on an in-class assessment
- I’d use AI to suggest what I should mark from my marking allocation based on how much time I have available
- I’d use AI to assist me while marking prose: To generate editable feedback on a rubric / marking criteria that AI wrote from my assessment & my prompt
- I’d use AI to assist me while marking prose: To generate editable feedback on a rubric / marking criteria that I (a human) have written
- I’d use AI to assist me while marking prose: My human generated feedback would be improved by the AI. (I could still edit again)
- I’d use AI to create adaptive assessments. Selecting the next question based on the answer of the previous question to maintain difficulty level
- I’d use AI to create an automatic retake for a candidate based on the questions they got wrong, with different questions of the same level
-
Below we explore their responses.
AI for Generating Non-MCQs
Question: I’d use AI to generate non-MCQs
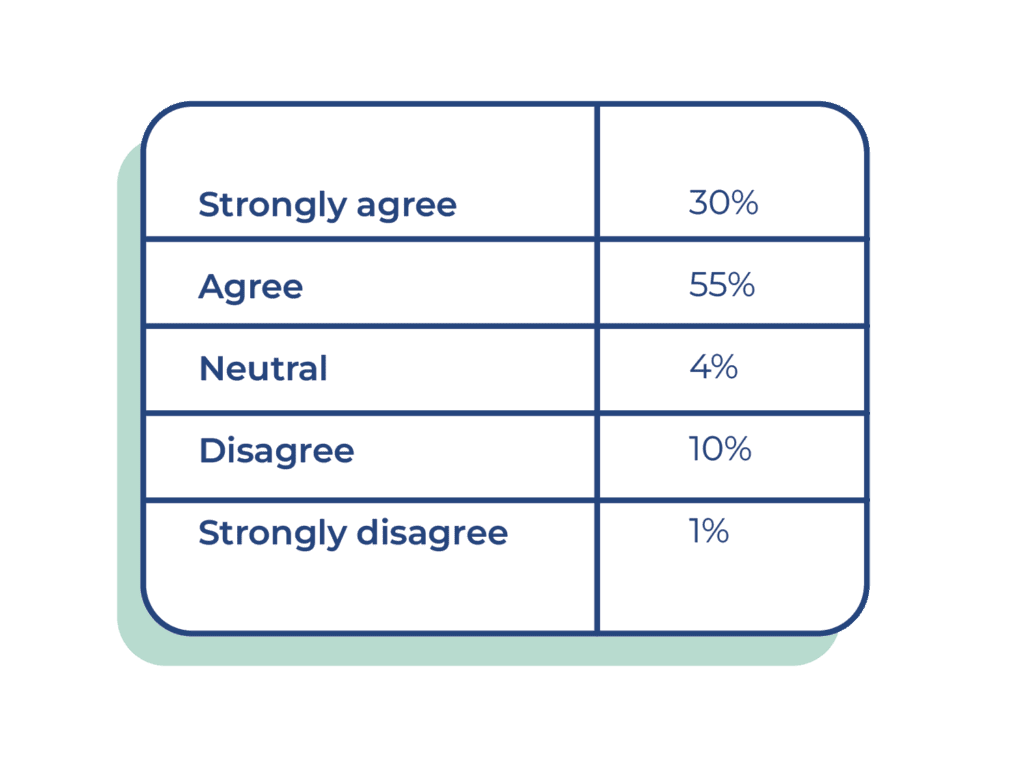
One of the most significant areas of interest was using AI to generate questions within the context of a syllabus or curriculum. Educators saw potential in AI’s ability to align generated questions with existing learning outcomes and course content. This was a strong preference over questions generated wholly by an LLM. The need for relevance and contextual accuracy is paramount.
Another application identified was the use of AI to create variations of a question stem or framework written by a human. For example, an educator might write the core idea or theme of a question, and the AI could generate alternative phrasings, formats, or scenarios to test the same concept in diverse ways. This capability could save time and support educators in designing assessments that cater to different levels or cohorts and to safeguard academic integrity.
In particular among those who wanted to use AI more gradually, an interest was expressed in AI’s ability to generate scenarios for assessment questions, with a preference for these scenarios to be tied to a human-authored question. Indicating a preference for AI to play a supportive role in content creation, rather than taking full responsibility for the task. By maintaining control over the core question, educators could ensure the quality and appropriateness of the assessment while benefiting from the efficiency of AI in generating supporting content.
AI for Tagging Questions to Create a Taxonomy
Question I’d use AI to tag my (human generated) questions to create a taxonomy for an item bank
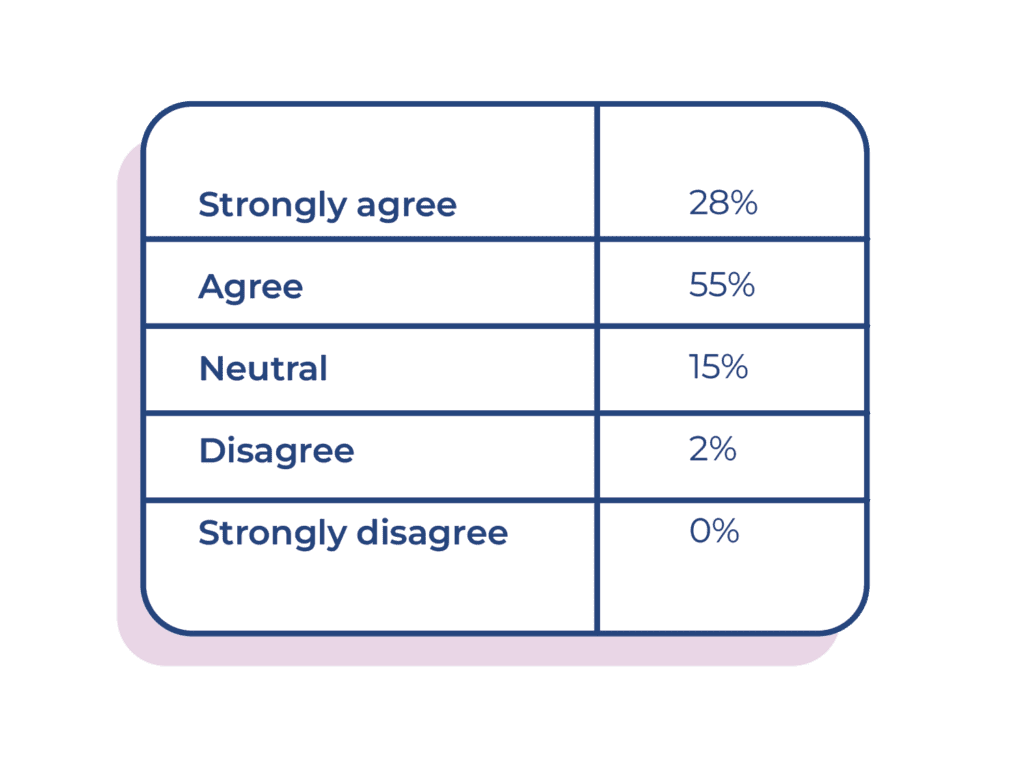
Educators showed interest in using AI to tag human-generated questions as part of creating a taxonomy for an item bank. The idea of using AI for tagging was welcomed on the basis of potential speed and efficiency.
Concerns that while AI might not be perfect were tempered by the idea it could provide a strong starting point or ‘first pass’ in the tagging process. This initial effort would allow educators to refine and improve the results without needing to start from scratch. By automating the more repetitive elements of taxonomy creation, AI has the potential to enable educators to focus on higher-order tasks of question quality and alignment with learning outcomes.
There was also a notable sense of comfort with AI working on human-generated content in this context. Educators viewed this use of AI as an expected use case. However, questions were raised about the nature of the process itself. Some respondents wondered whether AI tagging questions was truly innovative or simply an automated version of keyword extraction, a process computers have handled for years. Others wanted to understand what AI could do beyond what a human might achieve, aside from offering faster results. These concerns reflect a desire for clarity about the added value AI brings and an assurance that it is genuinely improving processes rather than merely accelerating them. Noting that acceleration has a value too, but accurately labeling what AI brings is important for buy-in.
AI for Dry-Running Assessments
Question: I’d use AI to ‘dry run’ an assessment I have written to give me a range of possible student answers
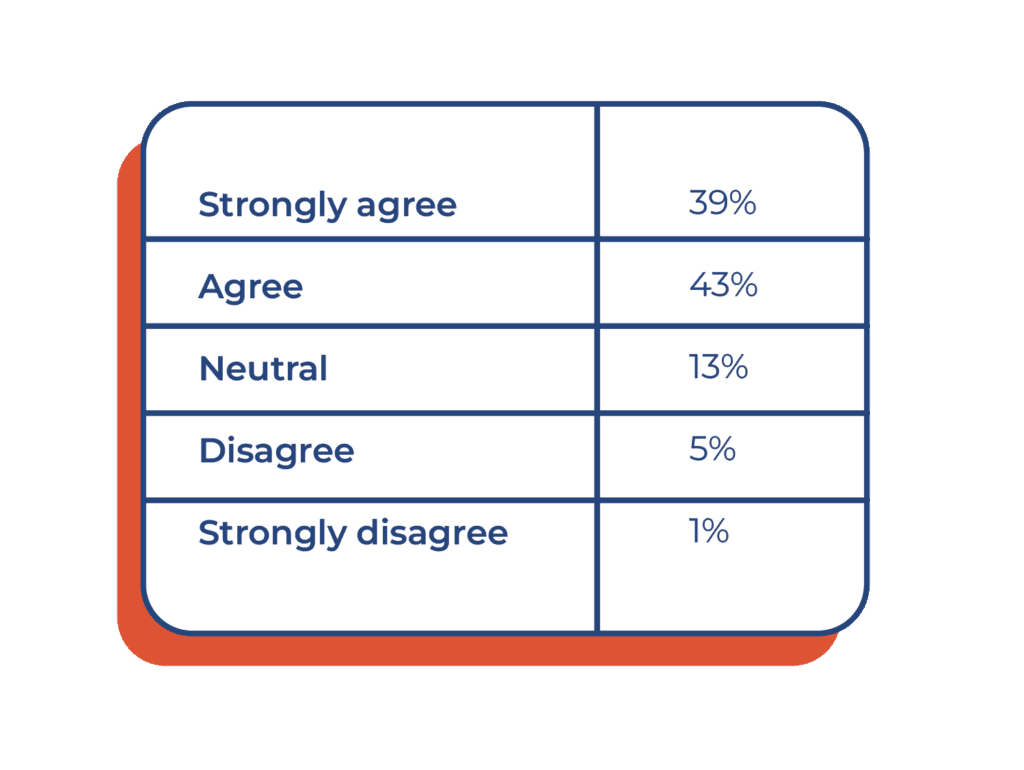
While generally agreeing with the proposition, educators expressed mixed views about using AI to ‘dry run’ assessments they have written by generating a range of possible student answers. They were skeptical whether AI can truly mimic a student’s reasoning or understanding, noting the idiosyncrasies of the range of student answers is beyond current capabilities. The prospective insights were how AI performs, not students.
One area of interest was evaluating how AI would respond to a question to determine its susceptibility to generative AI tools. Educators see a value in testing their assessments against AI-generated responses to identify how generative AI would answer it. By understanding how an AI might answer, educators could refine their questions to make them more robust and less predictable for AI systems. This could ensure that the assessments remain a genuine measure of student understanding rather than a test of their ability to navigate generative AI.
AI for Analyzing Data on Assessment Questions and Student Responses
Question: AI for Analyzing Data on Assessment Questions and Student Responses
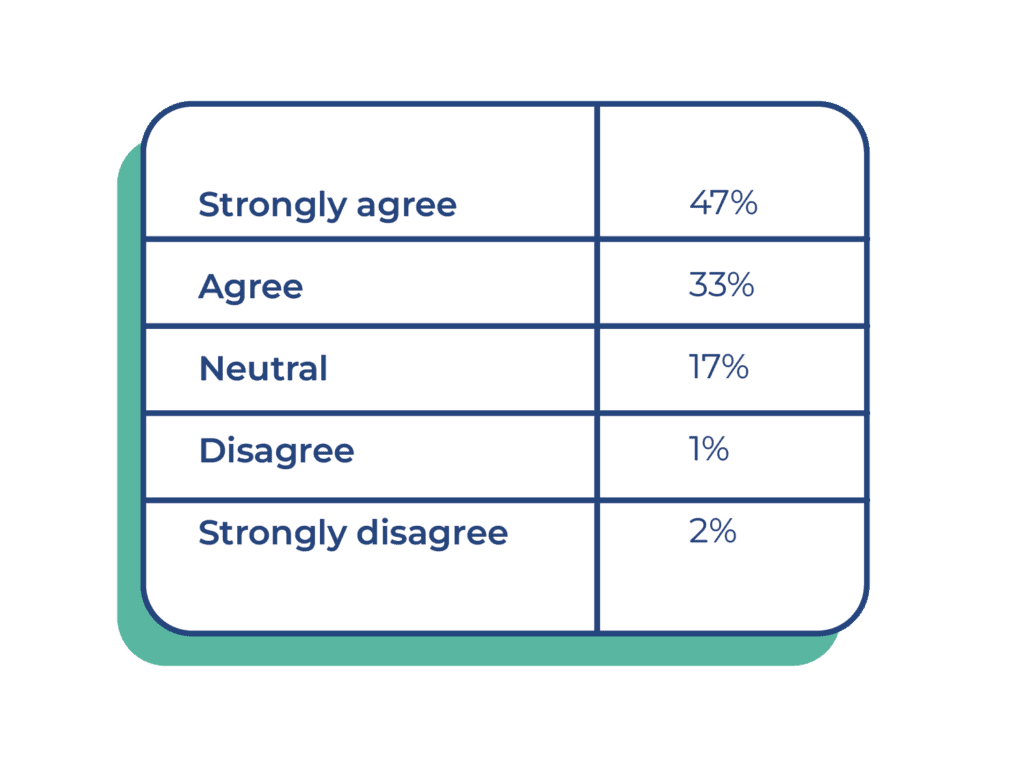
Educators expressed strong interest in leveraging AI to analyze data related to assessment questions and student responses. This application of AI aligns closely with their expectations of what the technology should be used for, namely handling complex data analysis tasks that would otherwise consume significant time and resources.
This was seen as a normal and valuable use of AI because it focuses on improving efficiency. Unlike applications of AI that might influence the teaching process, this use is viewed as supportive, offering insights that educators can use to refine their assessments and teaching strategies. By analyzing patterns in student responses, for example, AI can help identify trends, such as common misconceptions or areas where students consistently perform well. This data could inform future curriculum adjustments or targeted interventions.
There is also enthusiasm about the potential for AI to uncover insights that might not be quickly or efficiently available via manual analysis. For instance, AI could highlight correlations between question structure and student performance in real time to inform reuse.
However, some skepticism remains about what AI brings to this process beyond speed. Educators want clear evidence of how AI’s analysis goes beyond traditional statistical tools or manual review. Understanding the insights from AI, for example its ability to process large datasets quickly or detect patterns humans might overlook is essential for building confidence in its deployment.
AI for Generating Follow-Up Activities Based on In-Class Assessments
Question: I’d use AI to generate follow-up activities for students based on an in-class assessment
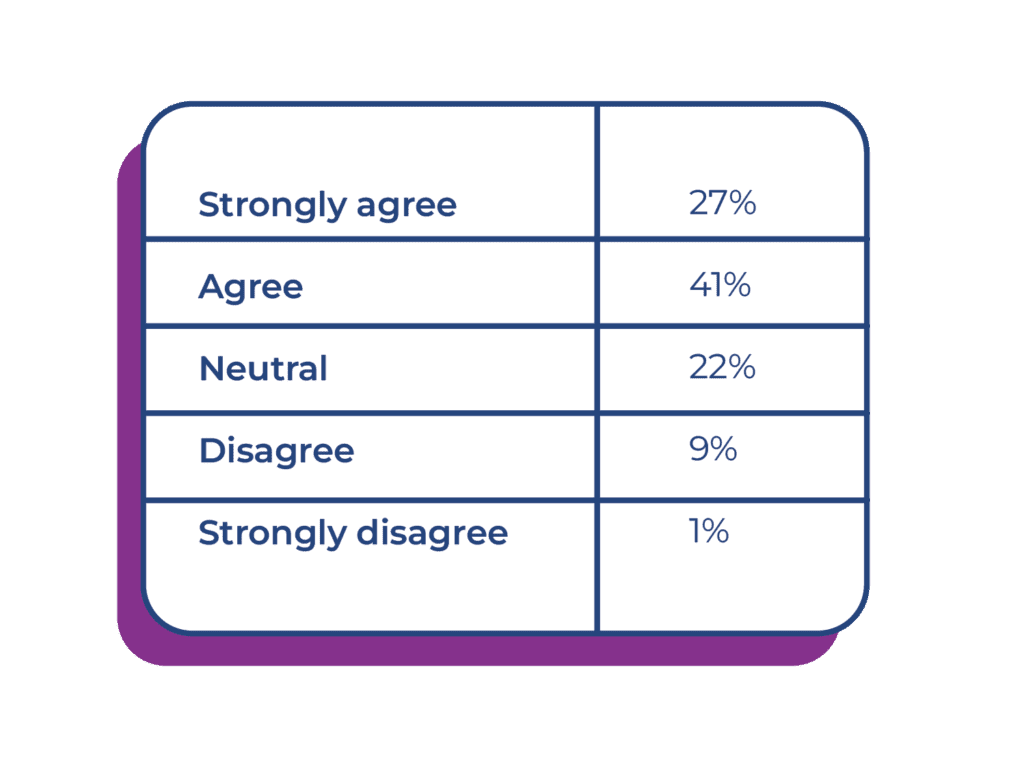
A key sentiment among educators was that similar tools already exist. Many wondered how AI would advance beyond what current systems offer. For instance, could AI provide more personalized or contextually relevant follow-up activities? Educators want to understand the benefits of AI in this space, especially in terms of its ability to improve upon existing functionality or introduce new capabilities.
Another common question was about the input required for AI to be effective. Educators highlighted that the utility of AI in generating follow-up activities depends heavily on the quality and quantity of the content provided. Without sufficient data or a clear framework, the AI’s output might lack the depth or relevance needed to engage students effectively. This raises concerns about the workload required to prepare materials for AI systems versus the time saved through automation.
Despite these questions, the potential for AI to assist in this area was recognised. Educators noted that AI could help by providing a starting point or template for follow-up activities, which they could then adapt and refine. This aligns with their broader preference for AI tools that support and enhance their work, rather than attempting to replace their expertise or judgment.
AI for Suggesting Marking Allocations Based on Time Availability
Question: I’d use AI to suggest what I should mark from my marking allocation based on how much time I have available
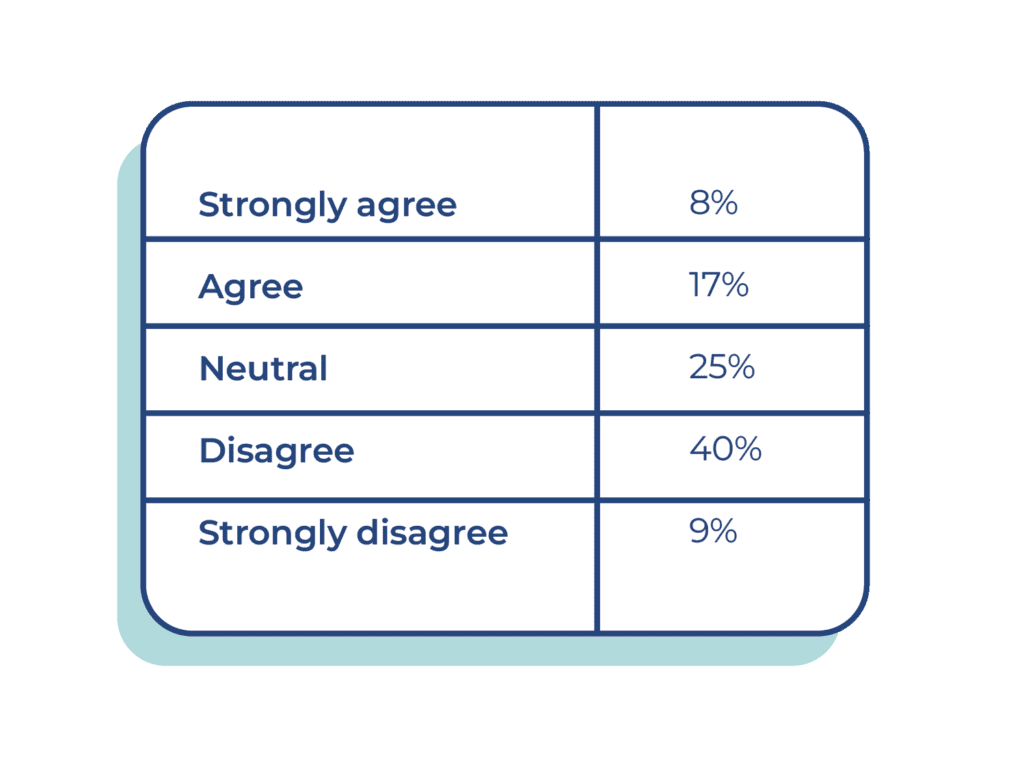
Educators expressed significant skepticism about using AI to suggest marking allocations based on how much time they have available. While the idea may seem efficient in principle, many questioned its practical value, citing concerns about its inability to account for individual marking patterns and behaviors.
A key concern was the perceived lack of depth in AI’s understanding of their marking practice. Educators felt that their approach to marking varies significantly depending on the nature of the task, the complexity of the questions, and the specific needs of their students. They doubted that AI could effectively predict these nuances and feared that its recommendations might reduce the quality of feedback provided to students.
Another apprehension was rooted in the potential for AI to standardize marking allocations based on the ‘quickest’ or most time-efficient methods. This raised concerns about a ‘lowest common denominator’ approach, where academics might be encouraged to mark within time constraints dictated by AI rather than focusing on providing detailed, thoughtful feedback. Such an approach, they argued, risks compromising the quality of the assessment process and undervaluing the role of educators in ensuring meaningful student learning experiences.
The possibility of reduced academic autonomy also surfaced in the discussions. Many felt that relying on AI for marking allocations could inadvertently erode their professional judgment and discretion, two elements central to their role as educators. There was also unease about whether AI might eventually be used to enforce institutional efficiency goals at the expense of academic rigor and personalization.
AI-Assisted Marking for Prose: Editable Feedback from AI or Human-Written Rubrics
First Question: I’d use AI to assist me while marking prose: To generate editable feedback on a rubric / marking criteria that AI wrote from my assessment & my prompt.

Second Question: I’d use AI to assist me while marking prose: To generate editable feedback on a rubric / marking criteria that I (a human) have written.

Educators expressed contrasting views about using AI to assist with marking prose, specifically regarding whether the AI-generated feedback should originate from an AI-created rubric or a human-authored one.
When the rubric or marking criteria were authored by AI, many educators felt uneasy, with a significant proportion disagreeing with the idea. The primary concern was that this approach delegated too much responsibility to AI, replacing educators in a critical aspect of assessment. For many, the creation of a rubric is central to the process of understanding and evaluating student work, and delegating this step to AI was seen as compromising the educator’s role. Some respondents felt that using AI in this way shifted the focus too far from education as a human-centered endeavor to an automated process.
Conversely, when the rubric or marking criteria were human-authored, there was much greater acceptance of AI playing a supporting role. Educators saw potential in using AI to provide a ‘first pass’ for summative assessments, particularly in tasks like generating editable feedback or highlighting patterns in student responses. However, even in this case, they were clear that AI should not make determinative judgments. The feedback generated by AI would need careful review and customization to ensure it aligns with the educator’s expectations and the unique needs of each student.
There was also notable interest in using AI to assist with formative assessments. In these cases, educators saw value in AI helping to provide quick, preliminary feedback, especially for large classes where personalized feedback might otherwise be delayed. AI’s ability to process data quickly and provide initial insights was recognized as a potential time saver, as long as the educator retained ultimate control over the quality and delivery of feedback.
Across both scenarios, those who agreed with the use of AI emphasized its role as an assistant rather than a replacement for educators.
AI for Improving Human-Generated Feedback During Marking
Question: I’d use AI to assist me while marking prose: My human-generated feedback would be improved by the AI. (I could still edit again)
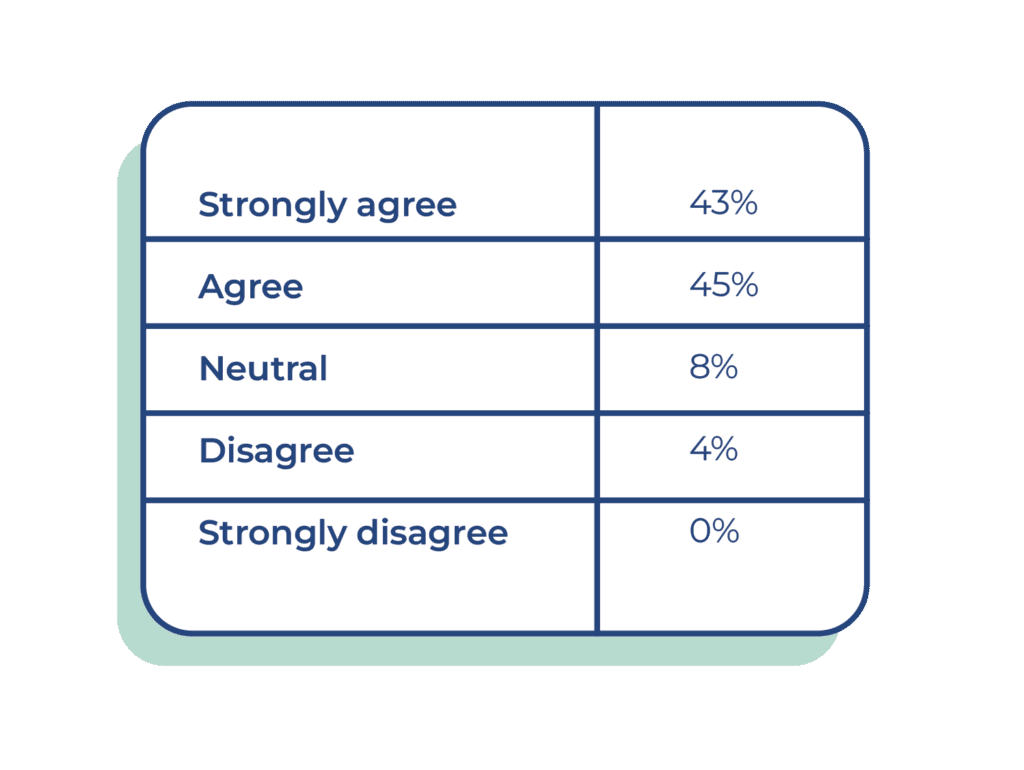
Educators showed considerable interest in using AI to improve human-generated feedback during marking, with many seeing value in its ability to refine, enhance, and align their comments with educational standards. However, this interest was accompanied by critical questions about AI’s capabilities and its potential impact on the authenticity of their feedback.
A potential method of making this more appealing would be if AI had the ability to link feedback directly to syllabus content or learning outcomes. Given the value of feedback aligned with learning outcomes, syllabus and curriculum, AI could help make these connections more explicit and consistent, supporting students’ understanding.
AI’s potential to enhance the tone and completeness of feedback was another widely recognized advantage. Some educators highlighted how AI might help soften overly critical comments or ensure that feedback is constructive and supportive. Additionally, AI could fill in gaps by suggesting areas for further feedback, ensuring that students receive comprehensive responses to their work.
Despite these potential benefits, educators raised concerns about how “machine-like” AI-generated feedback might sound. There were questions about whether AI could replicate the authentic voice of an academic, which many view as a key element of meaningful feedback. This concern reflects a broader hesitation about using AI in a way that might depersonalize the educator-student relationship.
AI for Creating Adaptive Assessments
Question: I’d use AI to create adaptive assessments. Selecting the next question based on the answer of the previous question to maintain difficulty level:
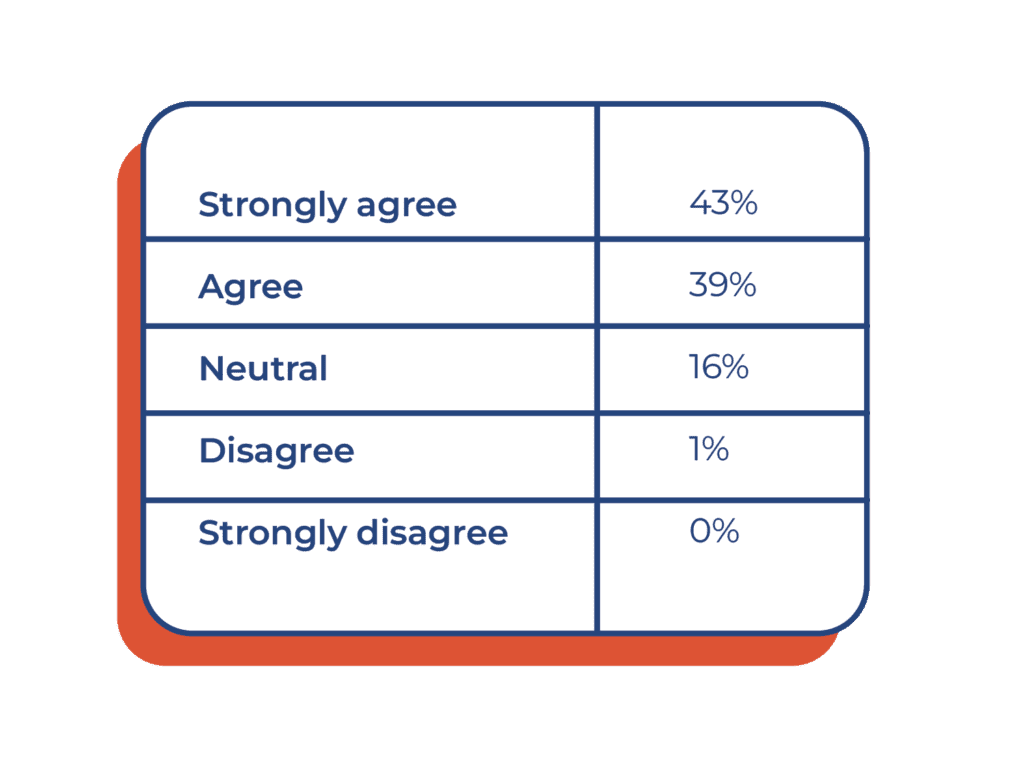
Educators expressed interest in using AI to create adaptive assessments that select subsequent questions based on student responses to maintain an appropriate difficulty level. While this concept garnered support, educators highlighted practical questions about its implementation and limitations.
One of the main points of interest was understanding how such adaptive assessments would work in practice. Educators were curious about the mechanisms AI would use to adjust question difficulty in real-time and how these decisions would be communicated transparently to both educators and students. This transparency was seen as essential for building trust in the system and ensuring that the adaptive process aligns with pedagogical goals.
Another significant concern was the data and content requirements for adaptive assessments. Educators questioned how much existing data on student performance and how many questions would be necessary to make the system effective. Without a sufficiently large and diverse question bank, there is a risk that the AI could generate inappropriate or repetitive questions, undermining the assessment’s value.
AI for Automatic Retake Generation
Question: I’d use AI to create an automatic retake for a candidate based on the questions they got wrong, with different questions of the same level
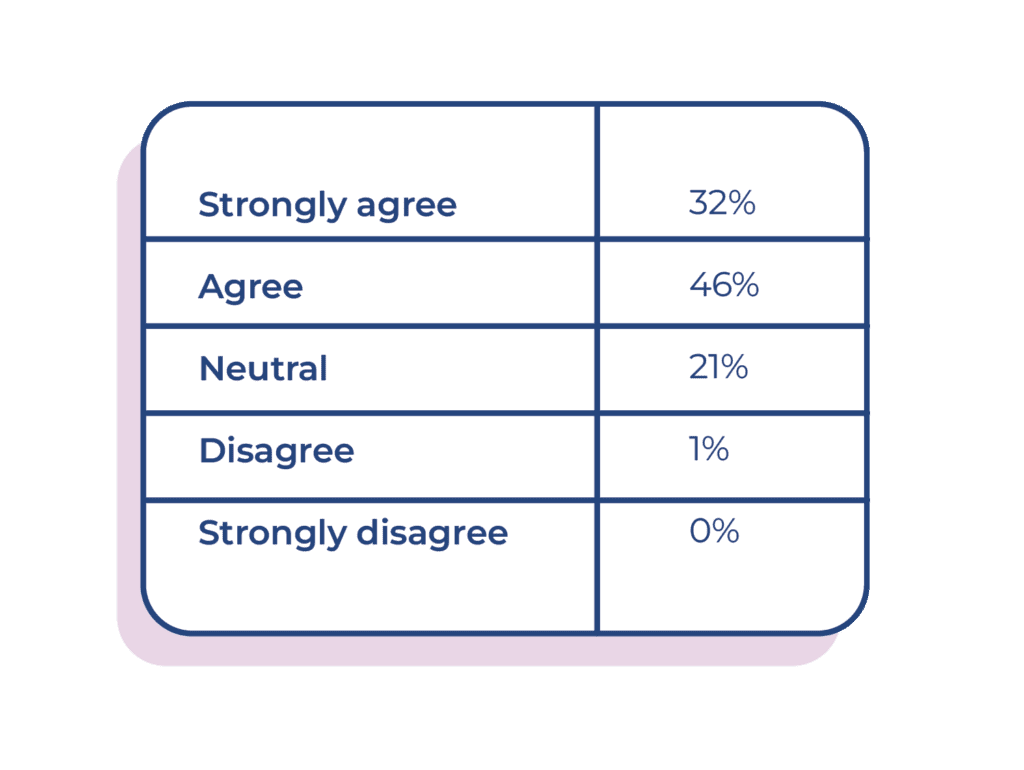
Educators showed interest in using AI to create automatic retakes for candidates, where new questions are generated based on the questions they initially answered incorrectly. This approach appealed particularly to those working under academic or professional regulations that require or prefer retake exams to feature different questions from the original assessment.
The ability of AI to automate this process was seen as a potential time saver, reducing the manual effort needed to construct retake exams while ensuring compliance with institutional policies. However, educators raised practical concerns about how much effort would be required to prepare a sufficiently large and diverse question bank, with level categorization, to support this functionality. Without a robust database of questions, the quality of AI-generated retakes could suffer, potentially compromising their educational value. Noting however, that in a non-AI environment, the same resource requirement is still present.
The Future of AI in Education
The valuable qualitative and quantitative insights gained from spending time with educators across the globe revealed the nuance in what they want from AI. Interest is far stronger in administrative efficiency and data analysis. Perhaps unsurprisingly, the closer AI gets to pedagogy, the more cautious the academy is to adopt it. The spectrum of concern ranges from ‘too much AI in the process’ to ‘what is AI adding to the process’ and several shades between.
Not every educator wants to use AI, nor should they have to. Like any tool, it has a purpose which needs to align to the pedagogy and context of the educator who uses it. Our guiding principle of pedagogy before technology is carried through into our approach on artificial intelligence. Educators need to trust in what they are using, to use it.
To find out more about how we see the future, download our Trustworthy AI white paper.